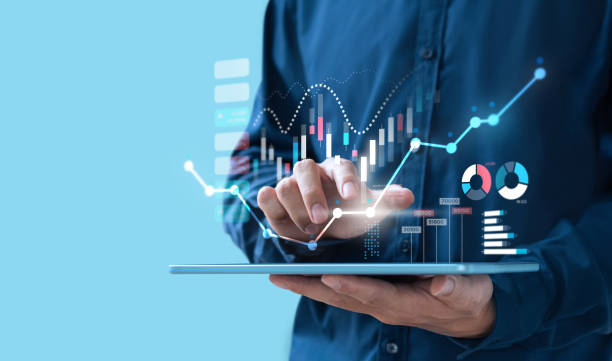
Clinical research is a critical component of advancing medical knowledge and patient care. However, this complex field is often intertwined with various statistical methods, employed to ensure the validity and reliability of research findings. Understanding these methods is vital, not just for researchers, but also for clinicians, allied health professionals, and even patients, as they navigate the world of medical research. In this blog, we’ll explore some of the common statistical methods used in clinical research.
1. Descriptive Statistics
The foundation of any statistical analysis, descriptive statistics summarize and organize the features of a dataset, providing a snapshot of its main characteristics. Descriptive statistics include measures of central tendency (mean, median, and mode), which indicate the center of a data distribution, and measures of dispersion (range, variance, standard deviation), which reflect the spread of data.
For instance, in a clinical trial, the mean age of participants, the median duration of treatment, or the standard deviation of patient weight all fall under descriptive statistics. These basic metrics provide a preliminary understanding of the data at hand.
2. Inferential Statistics
While descriptive statistics provide an overview of the data, inferential statistics let researchers make educated guesses (inferences) about a larger population based on the data from a smaller sample. In other words, inferential statistics make it possible to extrapolate findings from the study sample to the general population.
Key methods in inferential statistics include:
- Hypothesis Testing: It is used to make inferences or predictions about a population. The researcher starts with a null hypothesis (e.g., “Treatment A has no effect on disease B”) and an alternative hypothesis (e.g., “Treatment A affects disease B”). The data is then used to assess whether to accept or reject the null hypothesis.
- Chi-Square Test: This test is used to determine whether there’s a significant association between two categorical variables. For example, a chi-square test might be used to examine the relationship between disease incidence and diet (vegetarian vs. non-vegetarian).
- T-tests and ANOVA (Analysis of Variance): Both are used to compare means. A t-test is used when comparing the means of two groups, while ANOVA is used for three or more groups. For instance, researchers might use ANOVA to compare the mean blood pressure levels of patients across different treatment regimens.
3. Regression Analysis
Regression analysis investigates the relationship between a dependent (outcome) variable and one or more independent (predictor) variables. For instance, a simple linear regression might explore the relationship between dosage of a drug (independent variable) and patient recovery rate (dependent variable).
In cases where the outcome is a binary variable (e.g., the presence or absence of disease), logistic regression is used. It provides the odds ratio associated with a one-unit change in the predictor variable, offering valuable insights into risk factors.
4. Survival Analysis
Survival analysis is particularly important in clinical research for analyzing time-to-event data. It could be time until a patient’s death, relapse, or recovery. Techniques such as the Kaplan-Meier estimator (for estimating survival function) and Cox proportional hazards models (for examining the effect of predictor variables on survival) are widely used.
5. Non-parametric Methods
When data do not meet the assumptions required for parametric tests (e.g., normal distribution), non-parametric methods come into play. These tests, including the Mann-Whitney U test and the Kruskal-Wallis test, are less powerful but more flexible, making them useful for skewed distributions or ordinal data.
Power and Sample Size Calculations
Power analysis helps determine the minimum sample size required to detect an effect of a given size, which is crucial for designing robust clinical trials. Having a properly powered study ensures that if a significant difference or effect exists, the study is likely to detect it.
7. Multivariate Analysis
Multivariate analysis is used when dealing with more than one statistical outcome variable at a time. It provides a way to observe and analyze more than one statistical outcome variable at a time. Techniques such as multiple regression, multivariate analysis of variance (MANOVA), and multivariate regression are commonly used.
8. Meta-Analysis
Meta-analysis is a statistical technique for combining the findings from independent studies. This method is commonly used in systematic reviews and is valuable when there are multiple studies addressing the same research question, as it provides a more objective appraisal of evidence than traditional narrative reviews.
9. Bayesian Statistics
Bayesian statistics is a mathematical procedure that applies probabilities to statistical problems. It provides people the tools to update their beliefs in the evidence of new data. In the context of clinical trials, Bayesian statistics can be used to combine prior information about parameters of interest with data collected during the trial to make statistical inferences.
10. Machine Learning
While traditional statistical methods are still widely used, machine learning techniques are gaining popularity in clinical research. They can handle large amounts of data and can be used for prediction, classification, and clustering tasks. Common techniques include decision trees, random forests, and neural networks.
Conclusion
The field of clinical research is vast and complex, with various statistical methods adding layers of insight into health outcomes and the efficacy of interventions. Understanding these statistical techniques enables a deeper interpretation of the results, leading to better-informed decisions in patient care.
Whether you’re a seasoned researcher, a healthcare professional, or a curious reader, having a grasp of these statistical methods is valuable. It’s like learning a new language that opens up a world of information, helping us translate numbers into meaningful health outcomes.
Cloudbyz EDC is a user-friendly, cloud-based solution that is designed to store and manage clinical data effectively throughout a clinical trial’s life cycle. Our innovative solution enables clinical research teams to efficiently collect, analyze, and manage clinical data of different complexity and size. Cloudbyz EDC is a scalable solution and meets all the essential regulatory compliance requirements such as FDA- 21 CFR Part 11, GCP, GAMP5, HIPAA, and EU- GDPR.To know more about Cloudbyz EDC Solution contact info@cloudbyz.com